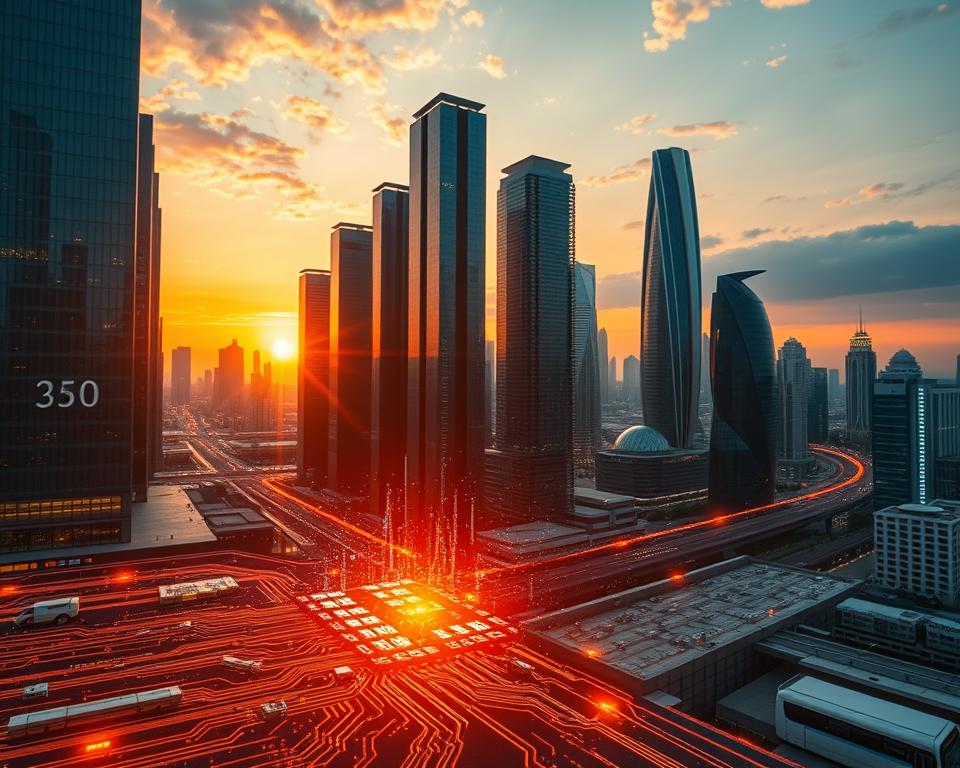
The Main Applications of Deep Learning in Today’s Tech
Did you know that the deep learning market is projected to reach a staggering $126 billion by 2025? This exponential growth underscores the impact of deep learning on technology across various sectors. As a subset of artificial intelligence, deep learning mimics the way our brains work, enabling machines to analyze vast amounts of data effortlessly. From revolutionizing healthcare with advanced diagnostics to enhancing customer experiences in retail, the question of what are the main applications of deep learning in today’s technology reveals its omnipresence in our daily lives. Its applications extend into finance with fraud detection and algorithmic trading, making it a transformative force we’re just beginning to understand.
Key Takeaways
- The deep learning market is expected to reach $126 billion by 2025.
- Deep learning mimics human brain functions for data analysis.
- Applications of deep learning span multiple domains, including healthcare and finance.
- Fraud detection and customer service improvements are key uses in various industries.
- Understanding deep learning is crucial for leveraging its benefits in modern technology.
Understanding Deep Learning
In the introduction to deep learning, it is essential to grasp the core concept of deep learning technology, which revolves around the use of neural networks. These networks consist of interconnected nodes or neurons, mimicking the human brain’s functionality. Unlike traditional machine learning models that rely heavily on manual feature extraction, deep learning automates this process. This automation plays a vital role in handling complex datasets with minimal human intervention.
At the heart of neural networks are layers, including input, hidden, and output layers. Each layer is responsible for different tasks in the learning process. Activation functions, such as ReLU or sigmoid, determine whether a neuron should be activated based on the input it receives. This decision-making capability allows the network to learn patterns and make predictions more effectively.
Another crucial aspect of deep learning is the mechanism of backpropagation. This process enables the model to adjust weights and biases based on the error in predictions, leading to improved accuracy over time. By iteratively refining its parameters, the neural network becomes more adept at recognizing patterns and relationships within the data.
Deep Learning in Computer Vision
Deep learning in computer vision has transformed how machines process visual information. This technology enables advanced capabilities in image recognition and classification, allowing systems to identify objects, faces, and scenes with remarkable accuracy. The integration of cutting-edge algorithms has made image recognition technology a vital tool across various industries.
Image Recognition and Classification
Image recognition involves training models to analyze visual data and categorize it effectively. These models utilize large datasets to learn distinct features of images. Applications span across multiple fields, from social media, where platforms like Facebook use this technology to recognize faces, to healthcare, utilizing deep learning for diagnosing medical conditions. The impact of image recognition on efficiency and accuracy in these domains cannot be overstated.
Object Detection and Tracking
Object detection uses deep learning techniques to identify and locate objects within images or video feeds. This capability is crucial for applications in retail, where businesses employ it for security purposes and to gain insights into customer behavior. With the advancement of augmented reality (AR) and virtual reality (VR), object detection technology continues to evolve, offering immersive experiences by recognizing and tracking real-world objects.
Application Area | Example of Use | Benefit |
---|---|---|
Social Media | Face Recognition | Enhances user experience by automatically tagging friends. |
Healthcare | Medical Imaging | Improves diagnostic accuracy through better image analysis. |
Retail | Security Surveillance | Reduces theft and improves customer insights. |
AR/VR | Object Interaction | Creates engaging, interactive environments for users. |
Speech Recognition with Deep Learning
Speech recognition has gained remarkable momentum through advancements in deep learning technologies. The integration of artificial intelligence in understanding human language has significantly transformed natural language processing applications. This evolution empowers devices to interpret voice commands more accurately, allowing for efficient human-computer interaction.
Natural Language Processing (NLP)
Natural language processing applications thrive on the capabilities of speech recognition deep learning. By analyzing and comprehending vast amounts of spoken language data, systems can learn nuances in tone, pronunciation, and context. This deep learning approach enhances the ability to transcribe speech accurately and deliver personalized responses, improving user satisfaction and engagement.
Voice Assistants and AI Communication
Popular voice assistants like Siri and Google Assistant showcase the potential of AI communication. These platforms rely on sophisticated deep learning models to understand queries and provide relevant information. By employing speech recognition deep learning, these assistants continuously improve, adapting to users’ unique speech patterns. Such technology creates a seamless, conversational experience, bridging the gap between humans and machines.
Deep Learning in Healthcare Technology
The transformative impact of deep learning in healthcare is evident in various sectors, particularly in medical imaging and drug discovery. By leveraging advanced algorithms, healthcare professionals can achieve enhanced accuracy and efficiency in diagnosing diseases and developing new treatments.
Medical Imaging and Diagnostics
Deep learning in healthcare has revolutionized medical imaging technology, providing tools that significantly improve diagnostic accuracy. Algorithms analyze images from MRI and CT scans, identifying patterns that might escape the human eye. This technology not only enhances the speed of diagnosis but also reduces the likelihood of errors, enabling healthcare providers to offer timely and effective treatments.
Drug Discovery and Development
The drug discovery processes have also benefited immensely from deep learning applications. By utilizing vast datasets, machine learning models can quickly predict which compounds may lead to successful drug candidates. This capability accelerates the identification of potential treatment options, effectively minimizing costs and time associated with traditional drug development methods. As a result, the pharmaceutical industry can bring innovative therapies to market more efficiently.
The main applications of deep learning can be observed across various sectors, influencing the way businesses operate and consumers interact with technology. In healthcare, deep learning aids in medical imaging and diagnostics, improving accuracy and speed in disease detection. The tech industry uses deep learning extensively for natural language processing, enabling sophisticated communication through voice assistants and chatbots.
Finance is another area benefiting from deep learning, with applications in fraud detection and algorithmic trading, allowing for enhanced decision-making and risk assessment. In the retail sector, companies utilize deep learning to personalize customer experiences, optimizing choices and recommendations for users based on their behavior.
Deep learning plays a significant role in autonomous systems, particularly in self-driving cars, where it enhances navigation and safety features. Finally, in the realm of entertainment, deep learning facilitates content recommendations, enriching user engagement with targeted suggestions.
Deep Learning Applications in Finance
Deep learning in finance has significantly transformed various aspects of the industry, offering innovative solutions for complex challenges. The adoption of cutting-edge technologies allows financial institutions to enhance their operational efficiency and decision-making processes. Two critical areas where deep learning excels include fraud detection and algorithmic trading.
Fraud Detection
Fraud detection technology has evolved dramatically with the integration of neural networks. By analyzing transaction data, deep learning models can swiftly identify unusual patterns that may indicate fraudulent activities. These systems leverage vast amounts of historical data, allowing them to learn and adapt to new fraud schemes. Financial entities employ these advanced tools to protect customers and minimize losses, achieving better accuracy compared to traditional detection methods.
Algorithmic Trading
Algorithmic trading strategies benefit immensely from deep learning capabilities. Traders use sophisticated algorithms that analyze extensive datasets to pinpoint trends and generate predictions for profitable trades. With the ability to process real-time information, deep learning systems enable firms to execute trades at optimal prices while minimizing risks. This data-driven approach enhances trading performance and offers a competitive edge in the fast-paced financial markets.
Application Area | Deep Learning Contribution | Benefits |
---|---|---|
Fraud Detection | Pattern recognition in transaction data | Enhanced accuracy and reduced false positives |
Algorithmic Trading | Data analysis for predictive modeling | Improved trading efficiency and risk management |
AI Technology Uses Deep Learning in Retail
Deep learning in retail is reshaping how businesses engage with customers and manage operations. This innovative technology allows retailers to offer personalized shopping experiences tailored to individual preferences. By analyzing vast amounts of data, brands like Amazon and Walmart gain insights into consumer behavior, ultimately leading to increased customer satisfaction and loyalty.
AI applications in retail technology extend beyond personalization. They play a crucial role in inventory management by predicting trends and optimizing stock levels. Retailers can minimize waste while ensuring popular items are always available for shoppers. This efficiency not only elevates the shopping experience but also supports better financial performance.
Another significant aspect is customer analytics. Through deep learning, retailers can segment their audiences more effectively, allowing for targeted marketing efforts that resonate with specific demographics. As a result, brands leverage these insights to develop campaigns that attract and retain customers. The combination of deep learning in retail along with robust AI applications in retail technology represents a comprehensive strategy for enhancing business operations.
The Role of Deep Learning in Autonomous Vehicles
Deep learning in autonomous vehicles plays a vital role in transforming transportation into a safer and more efficient experience. These advanced technologies utilize a range of sensors and data inputs, enabling vehicles to navigate with precision and respond to dynamic environments.
Navigation and Path Planning
Effective navigation technology is essential for autonomous vehicles. Deep learning algorithms process data from cameras, LIDAR, and radar to create detailed maps of surroundings. This real-time processing facilitates path planning by identifying the best routes while considering traffic, road conditions, and obstacles.
Safety and Obstacle Detection
Safety detection systems significantly enhance the security of autonomous driving. By leveraging deep learning, vehicles can accurately detect and classify objects—pedestrians, cyclists, other vehicles—thereby reducing the risk of accidents. The ability to make instant decisions based on live data supports safer and more reliable journeys.
Deep Learning for Enhanced User Experiences
Deep learning technology plays a pivotal role in enhancing user experiences across various digital platforms. Companies are leveraging these advancements to create tailored interactions that resonate with individual preferences. A prime example lies in personalized recommendation systems used by streaming giants like Netflix and Spotify. These platforms utilize deep learning algorithms to analyze user behavior and viewing habits, delivering content that aligns closely with personal interests.
AI-driven user interfaces make interactions more intuitive, streamlining navigation and engagement. By analyzing user data, these interfaces adapt in real-time to provide suggestions based on previous interactions, leading to a more satisfying experience. When users are presented with options that cater to their tastes, their likelihood of engagement increases significantly.
- Enhanced Content Delivery: Users receive personalized content tailored to their preferences.
- Improved Engagement: AI-driven user interfaces foster more interactive environments.
- Data-Driven Insights: Continuous learning from user interactions leads to better recommendations.
With the integration of deep learning into user experiences, businesses can better understand their audience and create solutions that not only meet needs but also enhance satisfaction. This powerful technology transforms how users interact with platforms, ultimately resulting in higher retention rates and growth for companies. As deep learning continues to evolve, its impact on user experience will only become more profound.
Real-Time Data Analysis Using Deep Learning
In today’s fast-paced environment, the demand for real-time data analysis deep learning solutions is skyrocketing. Businesses across various sectors are harnessing these technologies to process and analyze vast amounts of data instantaneously. This capability allows organizations to make informed decisions quickly, tapping into emerging market trends while responding to user sentiments efficiently.
Industries such as e-commerce, finance, and social media extensively utilize big data analytics to extract valuable insights from user activity and market fluctuations. For instance, online retailers can instantly understand customer preferences and modify offerings accordingly. Financial institutions leverage real-time analysis to detect fraudulent activities and respond proactively.
The convergence of deep learning with real-time data analysis transforms how businesses interpret information, driving competitive advantage. By implementing effective algorithms that can learn and adapt, organizations can enhance their decision-making processes, ultimately paving the way for innovation and growth.
Impact of Deep Learning on Technology
The impact of deep learning is evident across diverse technological sectors, shaping how industries innovate and operate. Through advanced algorithms and extensive data analysis, deep learning enhances productivity, automates processes, and enables smarter solutions that were not possible before.
One significant area affected by technological advancements with deep learning is manufacturing. Companies are leveraging deep learning to streamline operations, significantly reducing downtime and improving quality control. Predictive analytics allows businesses to anticipate equipment failures and address issues proactively, enhancing overall efficiency.
Healthcare also benefits from these advancements. Algorithms trained on vast datasets assist in diagnosing diseases more accurately than ever, leading to better patient outcomes. This shift towards data-centric medicine underscores the transformative potential of deep learning technology.
Additionally, the impact of deep learning can be observed in finance, where institutions utilize machine learning models to detect fraudulent activity. The speed and accuracy with which deep learning algorithms analyze transactions surpass traditional methods, promoting enhanced security and trust in financial systems.
Sector | Technological Advancements with Deep Learning | Impact |
---|---|---|
Manufacturing | Predictive maintenance | Reduced downtime, increased efficiency |
Healthcare | Improved diagnostics | Better patient outcomes, personalized treatment |
Finance | Fraud detection | Enhanced security, increased trust |
Retail | Customer data analysis | Targeted marketing, improved user experience |
The continual evolution of deep learning not only enhances existing processes but also opens doors for new innovations. Adopting this technology aligns businesses with modern demands, ensuring sustainability and competitive advantage in an ever-changing landscape.
Machine Learning Applications Today
In the current landscape, machine learning applications play a pivotal role across various sectors. Predictive analytics stands out as a key application, combining advanced data analysis techniques with deep learning algorithms to forecast future trends based on historical data. Businesses harness these insights to drive strategies that align with anticipated market movements.
Predictive Analytics
Predictive analytics deep learning utilizes complex algorithms to analyze patterns within large data sets. Organizations employ this technique for tasks ranging from customer behavior analysis to risk assessment and inventory prediction. A variety of industries benefit from its capabilities, tailoring solutions to meet their specific needs.
Industry | Application of Predictive Analytics | Benefits |
---|---|---|
Healthcare | Disease prediction and management | Improved patient outcomes through preventative care |
Finance | Fraud detection and credit scoring | Enhanced security and risk management |
Retail | Customer purchase forecasting | Optimized inventory and increased sales |
Marketing | Targeted advertising strategies | Better ROI through accurate audience targeting |
Modern Uses of Deep Learning in Robotics
Deep learning in robotics has sparked significant advancements in automation, leading to increased efficiency and precision in various sectors. The application of this technology enables robots to learn and adapt, streamlining processes that once required human intervention.
Intelligent Task Automation
Task automation with AI revolutionizes industries by employing robots to handle repetitive or complex tasks. These robots utilize deep learning algorithms to recognize patterns, make decisions, and continually improve their performance over time. Common applications include:
- Manufacturing: Robots equipped with deep learning algorithms enhance production lines, reducing human error and increasing output.
- Logistics: Automated systems manage inventory and optimize deliveries, leading to a more efficient supply chain.
- Service Delivery: From customer support to maintenance tasks, robots improve service speed and accuracy while minimizing operational costs.
The following table illustrates how different industries leverage deep learning in robotics for intelligent task automation:
Industry | Application | Benefits |
---|---|---|
Manufacturing | Assembly line automation | Increased production speed, reduced waste |
Logistics | Automated warehousing | Enhanced inventory management, cost efficiency |
Healthcare | Surgical assistance and patient care | Improved accuracy, better patient outcomes |
Retail | Checkout automation | Faster service, improved customer experience |
Future Trends in Deep Learning Technology
The future trends deep learning landscape promises exciting advancements that may redefine industries. One critical trend involves the evolution of unsupervised learning techniques, enabling models to draw insights from unlabeled data. This approach can significantly enhance machine learning’s capabilities, making it invaluable for numerous applications.
Another pivotal area lies in the intersection of deep learning and emerging technologies in AI, particularly quantum computing. As quantum machines continue to develop, they hold the potential to process vast datasets at unprecedented speeds. This capability can revolutionize deep learning algorithms, unlocking new possibilities and driving efficiency in real-time analytics.
Ethical considerations surrounding AI deployment are gaining attention, highlighting the need for responsible practices in deep learning. As AI systems become more integrated into daily life, ensuring that these technologies operate fairly and transparently becomes paramount.
The shifting landscape will likely influence various sectors, from finance to healthcare, pushing for solutions that prioritize not only effectiveness but also societal impact. Change is inevitable, and these trends can shape how deep learning technology integrates into our future.
Conclusion
In summary, the impact of deep learning on various technological fields has been nothing short of transformative. From revolutionizing healthcare diagnostics and enhancing autonomous vehicles to improving finance and retail experiences, deep learning technologies have reshaped how industries operate and interact with consumers. This summary of deep learning impact highlights the remarkable advancements achieved so far and underscores the importance of this technology in our daily lives.
As we look to the future of technology, it is clear that the advancements in deep learning will continue to push boundaries and lead to innovations we have yet to imagine. Emerging applications, whether in robotics, natural language processing, or real-time data analysis, promise to improve efficiencies and drive growth across different sectors. The potential for deep learning technologies to influence future trends is immense, setting the stage for a smarter, more integrated world.
With ongoing research and developments, we can expect deep learning to be at the forefront of technological innovation. Anticipating the next breakthroughs will be key as we navigate this exciting landscape, welcoming new opportunities and improvements that deep learning technology holds for our future.